Hyperbolic Image Segmentation
CVPR 2022
-
Mina Ghadimi Atigh
UvA -
Julian Schoep
UvA -
Erman Acar
Leiden University, Vrije Universiteit Amsterdam -
Nanne van Noord
UvA - Pascal Mettes UvA
Abstract
For image segmentation, the current standard is to perform pixel-level optimization and inference in Euclidean output embedding spaces through linear hyperplanes. In this work, we show that hyperbolic manifolds provide a valuable alternative for image segmentation and propose a tractable formulation of hierarchical pixel-level classification in hyperbolic space. Hyperbolic Image Segmentation opens up new possibilities and practical benefits for segmentation, such as uncertainty estimation and boundary information for free, zero-label generalization, and increased performance in low-dimensional output embeddings.
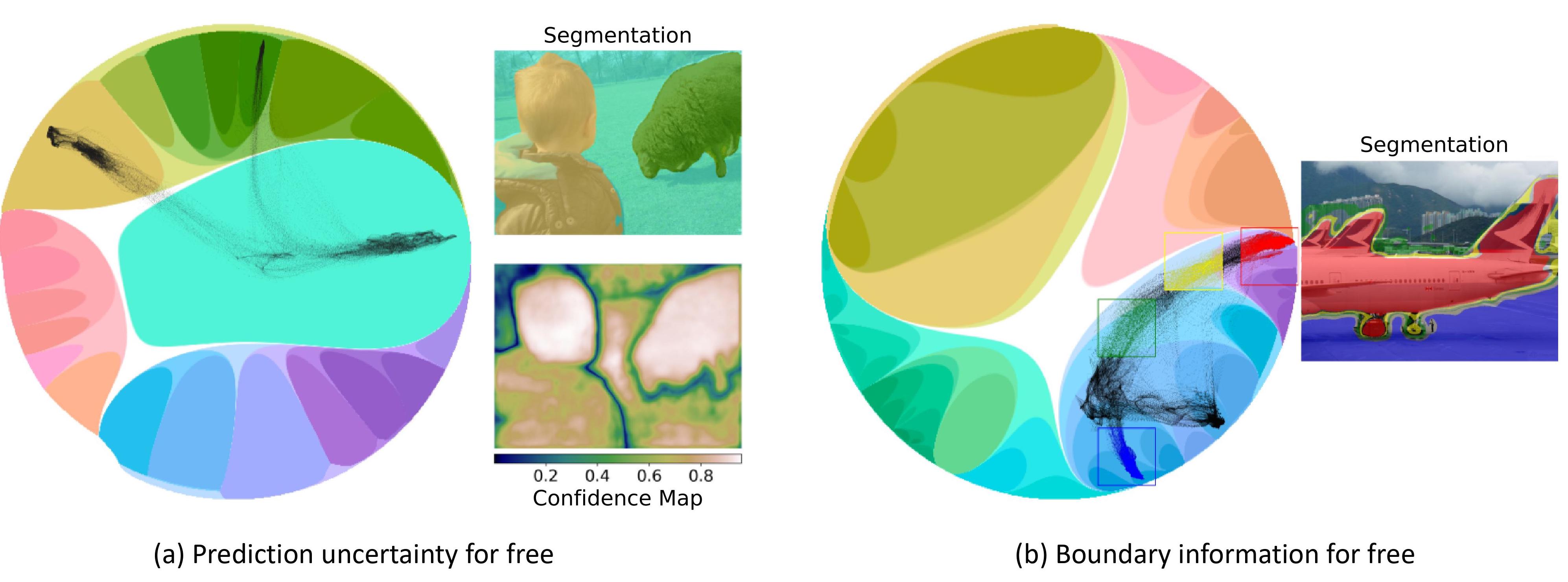